Digital Marketing - Study Notes:
What is Big Data?
Big Data describes the huge volume of data – both structured and unstructured – that inundates a business on a day-to-day basis. It involves datasets that are too large to store on one machine and it requires multiple computers to work together to process the volume of data. In turn, we can use the data to predict better results and forecasts than would be possible with smaller data sets.
Now, let’s think about where Big Data has come from, and some of the key associated technologies around it. We can now mesh different datasets to create core insights and understanding about our customers and environment better than we’ve could before - it’s the coming together of a number of different tools and techniques. Some of the key technologies in this area are around predictive analytics - being able to use the data to understand how things may happen in the future.
Big Data technologies
Some technologies associated with Big Data include:
- Predictive analytics: using large data sets to model and outcomes based on historical data.
- NoSQL databases: These offer high operational speed and flexibility for data managers.
- Search and knowledge discovery: This involves using AI to help process and sequence data for knowledge discovery.
- Stream analytics: You can integrate external data sources to enable applications to integrate, update, and process additional data.
- In-memory data fabric: This provides a comprehensive view of business data from different sources working together through automatic orchestration.
- Distributed file stores: You can store files on a server for access by users across a wide network, for example.
- Data virtualization: This provides access to data without needing to know the technical details or where the data is stored, including how it is formatted, for example.
- Data integration tools: These unify data from multiple sources, giving a view of combined data.
- Data preparation software: This enables you to combine, structure, and organize data in preparation for data visualization, analytics, and machine learning.
- Data quality software: This helps you to optimize the quality of your data and deploy pre-built software to users to manage data use.
Predictive analytics
Predictive analytics is software and/or hardware solutions that enable firms to discover, evaluate, optimize, and deploy predictive models by analyzing Big Data sources to improve business performance or mitigate risk. Effectively, this is about making core predictions based on previous behavior to evaluate what we think is the likely outcome for the future.
This is very important when thinking about buyer behavior, for example, in a marketing world. We need to be intuitive about what exactly is going to happen, and understand the variables that have changed from the past in order to make certain assumptions about what might happen in the future. It’s also worthwhile using common sense and intuition when examining the data to add to the benefit of your experience of the metrics.
You can think of Big Data technologies as being like a jigsaw puzzle. It’s all about meshing technologies together. There’s an element of overlap, as well as linkages, because all these technologies need to come together to create a robust Big Data strategy. And if you don’t have these linkages, your picture might not be complete.
The four Vs
[1.3.1.4] When thinking about Big Data, you can use a number of different approaches. One very useful framework involves the four Vs:
- Volume: the size or scale of the data
- Variety: different forms of data
- Veracity: trustworthiness of the data
- Velocity: frequency of incoming data
Volume
Big Data, by its very nature, is a larger number of data sources, and can provide greater volumes of insights on individual customers. It’s the aggregating of the information that creates the richness within the dataset. In other words, it’s not just a single dataset. It’s about meshing a number of different data sources.
Variety
Big Data includes a wider variety of data types and sources. For example, the data could be a rich combination of structured, semi-structured, and unstructured data.
Veracity
Veracity refers to the need to have robust data. Essentially, you must have some form of integrity within your data. Make it trusted, make it clean, and make it de-duplicated, because any anomalies that you have within it will eventually lead to inaccuracies that you might not notice later on. Remember, robust inputs lead to robust outputs, and this applies to Big Data as well.
Velocity
This is the frequency of new data being entered into the set. It’s always better to work with fresh data and data sets with high levels of data velocity.
Back to TopJack Preston
Jack Preston is a Data Scientist working within marketing analytics, with a particular focus on strategic customer loyalty. Jack has experience working in both small-scale startups and large corporates, including dunnhumby and Notonthehighstreet. He also holds an MSc in Business Analytics from UCL where he graduated with distinction.
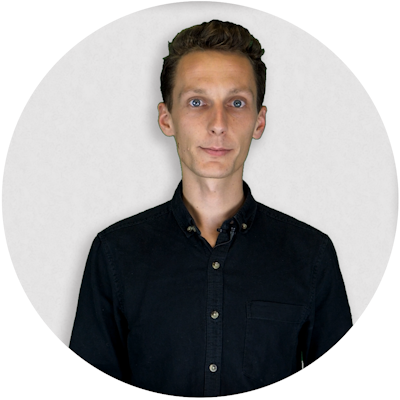